Anisotropic Diffusion in Image Processing
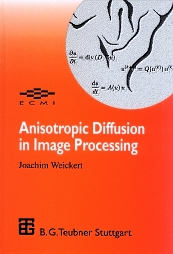
简介:
图像处理中的各向异性扩散:一种智能的平滑算法
各向异性扩散是一种在图像处理中广泛应用的自适应平滑技术。与传统高斯滤波器等各向同性扩散方法不同,它能够根据图像局部特征调整扩散方向和强度,在去除噪声的同时有效保护图像边缘信息。
该方法的核心思想是:在图像的不同区域施加不同的扩散系数。具体而言,算法会在每个像素点处计算其梯度方向,并沿着梯度方向进行抑制,而与梯度方向垂直的方向则保持较低的扩散率。这种选择性扩散策略使得平滑过程更加智能。
各向异性扩散在计算机视觉领域具有重要应用价值。它不仅能够有效去除图像噪声,还能较好地保持图像边缘、角点等重要特征信息,为后续图像分析和理解任务提供高质量的数据基础。近年来,随着深度学习的兴起,各向异性扩散的思想也被融入到卷积神经网络中,推动了图像处理技术的发展。
总体而言,各向异性扩散是一种兼具智能性和实用性的图像平滑算法,在噪声抑制与细节保护之间找到了良好平衡点,为图像处理领域提供了重要工具。
英文简介:
PDE-based image processing techniques are mainly used for smoothing and restoration purposes. Many evolution equations for restoring images can be derived as gradient descent methods for minimizing a suitable energy functional, and the restored image is given by the steady-state of this process. Typical PDE techniques for image smoothing regard the original image as initial state of a parabolic (diffusion-like) process, and extract filtered versions from its temporal evolution. The whole evolution can be regarded as a so-called scale-space, an embedding of the original image into a family of subsequently simpler, more global representations of it. Since this introduces a hierarchy into the image structures, one can use a scale-space representation for extracting semantically important information.
One of the two goals of this book is to give an overview of the state-of-the-art of PDE-based methods for image enhancement and smoothing. Emphasis is put on a unified description of the underlying ideas, theoretical results, numerical approximations, generalizations and applications, but also historical remarks and pointers to open questions can be found. Although being concise, this part covers a broad spectrum: it includes for instance an early Japanese scale-space axiomatic, the Mumford–Shah functional for image segmentation, continuous-scale morphology, active contour models and shock filters. Many references are given which point the reader to useful original literature for a task at hand.
The second goal of this book is to present an in-depth treatment of an interesting class of parabolic equations which may bridge the gap between scale-space and restoration ideas: nonlinear diffusion filters. Methods of this type have been proposed for the first time by Perona and Malik in 1987 [326]. In order to smooth an image and to simultaneously enhance important features such as edges, they apply a diffusion process whose diffusivity is steered by derivatives of the evolving image. These filters are difficult to analyse mathematically, as they may act locally like a backward diffusion process. This gives rise to well-posedness questions. On the other hand, nonlinear diffusion filters are frequently applied with very impressive results; so there appears the need for a theoretical foundation.
- 书名
- Anisotropic Diffusion in Image Processing
- 译名
- 图像处理中的各向异性扩散
- 语言
- 英语
- 年份
- 1998
- 页数
- 184页
- 大小
- 3.17 MB
- 下载
Anisotropic Diffusion in Image Processing.pdf
- 密码
- 65536
最后更新:2025-04-12 23:58:12