Using R for Introductory Statistics
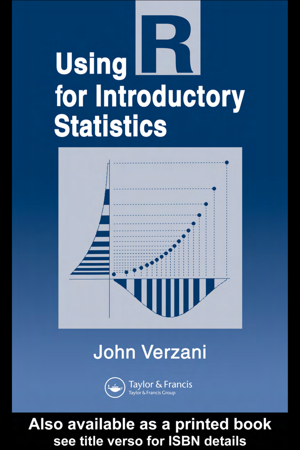
简介:
统计计算软件的成本使许多大学无法安装这些有价值的计算和分析工具。R是一个功能强大的开源软件包,是针对这个问题创建的。自引入以来,由于其连贯性,灵活性和免费可用性,它一直在爆炸式增长。虽然对于第一次学习统计学的学生来说,它是一个有价值的工具,但采用它需要适当的介绍性材料。
使用R进行入门统计填补了文献中的这一空白,使入门学生可以访问该软件。作者介绍了统计主题的独立处理和R软件的复杂性。节奏是这样的,学生能够掌握数据处理和探索,然后再潜入更先进的统计概念。这本书比典型的更关注探索性数据分析,包括模拟的一章,并提供了一个统一的线性模型方法。本文为R在统计学中的进一步研究和发展奠定了基础。附录涵盖了R的安装,图形用户界面和教学,以及有关编写功能和生成图形的信息。这是将统计研究与强大的计算工具集成在一起的理想文本。
英文简介:
The cost of statistical computing software has precluded many universities from installing these valuable computational and analytical tools. R, a powerful open-source software package, was created in response to this issue. It has enjoyed explosive growth since its introduction, owing to its coherence, flexibility, and free availability. While it is a valuable tool for students who are first learning statistics, proper introductory materials are needed for its adoption.
Using R for Introductory Statistics fills this gap in the literature, making the software accessible to the introductory student. The author presents a self-contained treatment of statistical topics and the intricacies of the R software. The pacing is such that students are able to master data manipulation and exploration before diving into more advanced statistical concepts. The book treats exploratory data analysis with more attention than is typical, includes a chapter on simulation, and provides a unified approach to linear models.
This text lays the foundation for further study and development in statistics using R. Appendices cover installation, graphical user interfaces, and teaching with R, as well as information on writing functions and producing graphics. This is an ideal text for integrating the study of statistics with a powerful computational tool.
- 书名
- Using R for Introductory Statistics
- 译名
- 使用 R 进行入门统计学
- 语言
- 英语
- 年份
- 2004
- 页数
- 413页
- 大小
- 2.99 MB
- 标签
- 统计学
- R语言
- 下载
Using R for Introductory Statistics.pdf
- 密码
- 65536
最后更新:2025-04-12 23:57:34