Interpretable Machine Learning, 2nd Edition: A Guide for Making Black Box Models Explainable
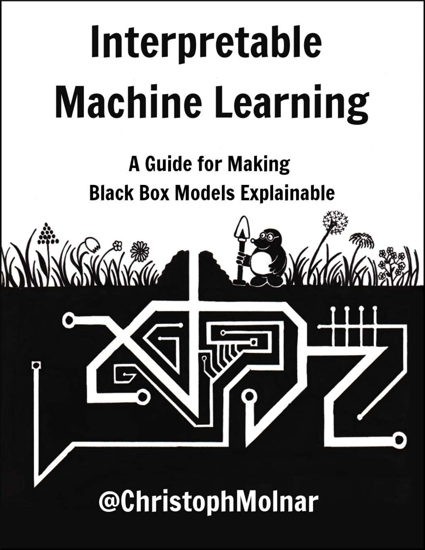
简介:
本书涵盖了一系列可解释性方法,从固有的可解释模型到可以使任何模型可解释的方法,例如SHAP,LIME和排列特征重要性。
英文简介:
TThis book covers a range of interpretability methods, from inherently interpretable models to methods that can make any model interpretable, such as SHAP, LIME and permutation feature importance.
- 书名
- Interpretable Machine Learning, 2nd Edition: A Guide for Making Black Box Models Explainable
- 译名
- 可解释机器学习,第二版:使黑盒模型可解释的指南
- 语言
- 英语
- 年份
- 2019
- 页数
- 251页
- 大小
- 3.45 MB
- 标签
- 机器学习
- 下载
Interpretable Machine Learning, 2nd Edition: A Guide for Making Black Box Models Explainable.pdf
- 密码
- 65536
最后更新:2025-04-12 23:55:06