Reinforcement Learning and Optimal Control
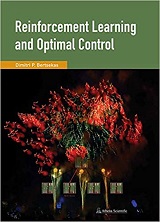
简介:
强化学习 (RL) 是人工智能中最活跃的研究领域之一,是一种计算学习方法,通过这种方法,智能体试图在与复杂、不确定的环境交互时最大化其获得的奖励总量。本书的目的是考虑大型且具有挑战性的多阶段决策问题,这些问题原则上可以通过动态规划和最优控制来解决,但是它们的精确解在计算上很难解决。
我们更多地依赖直观的解释,而不是基于证明的见解。我们仍然在附录中提供了有限和无限水平动态规划理论的严格简短说明,以及一些基本的近似方法。为此,我们需要适度的数学背景: 微积分,基本概率以及矩阵向量代数的最小使用。
英文简介:
Reinforcement Learning (RL), one of the most active research areas in artificial intelligence, is a computational approach to learning whereby an agent tries to maximize the total amount of reward it receives while interacting with a complex, uncertain environment.
The purpose of the book is to consider large and challenging multistage decision problems, which can be solved in principle by dynamic programming and optimal control, but their exact solution is computationally intractable.
We rely more on intuitive explanations and less on proof-based insights. Still we provide a rigorous short account of the theory of finite and infinite horizon dynamic programming, and some basic approximation methods, in an appendix. For this we require a modest mathematical background: calculus, elementary probability, and a minimal use of matrix-vector algebra.
- 书名
- Reinforcement Learning and Optimal Control
- 译名
- 强化学习与最优控制
- 语言
- 英语
- 年份
- 2019
- 页数
- 268页
- 大小
- 2.77 MB
- 标签
- 强化学习
- 机器学习
- 下载
Reinforcement Learning and Optimal Control.pdf
- 密码
- 65536
最后更新:2025-04-12 23:54:38
←Control Engineering: An Introduction with the Use of MATLAB